Multilingual Coarse Political Stance Classification of Media: Corpora Compilation
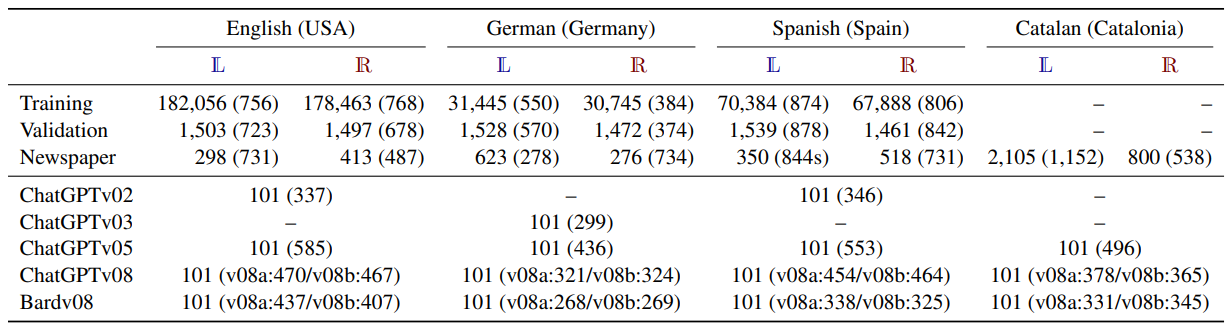
:::info
This paper is available on arxiv under CC BY-NC-SA 4.0 DEED license.
Authors:
(1) Cristina España-Bonet, DFKI GmbH, Saarland Informatics Campus.
:::
Table of Links
Abstract and Intro
Corpora Compilation
Political Stance Classification
Summary and Conclusions
Limitations and Ethics Statement
Acknowledgments and References
A. Newspapers in OSCAR 22.01
B. Topics
C. Distribution of Topics per Newspaper
D. Subjects for the ChatGPT and Bard Article Generation
E. Stance Classification at Article Level
F. Training Details
2. Corpora Compilation
We approach our task as a classification problem with two classes: Left (L) and Right (R) political orientations. This is a simplification of the real problem, where articles can also be neutral and there might be different degrees of biases. Previous work relied on 3 or 5 classes, always including the neutral option (Baly et al., 2020; Aksenov et al., 2021). In these works, data was manually annotated creating high quality training data but also limiting a lot the scope of the work in terms of languages and countries covered. When using the fine-grained classification scale, the authors acknowledge a bad generalisation of the classifiers to new sources. On the other hand, García-Díaz et al. (2022) and Russo et al. (2023) exclude the neutral class and work with a binary or multiclass Left–Right classifications of tweets from Spanish and Italian politicians respectively, but their work does not include longer texts. The binary classification might be justified as they worked with tweets, a genre where people tend to be more visceral and therefore probably more polarised. In our case, we need to be sure that the classifier generalises well to unseen sources and we stick to the 2-class task while minimising the number of neutral articles in training (see below).
\
Distant Supervision. As far as we know, only a manually annotated newspaper corpus in English (Baly et al., 2020) and another one in German (Aksenov et al., 2021) are available. We follow a different approach in the spirit of Kulkarni et al. (2018) and Kiesel et al. (2019). We do not manually annotate any article, but we trust AllSides, MB/FC, Political Watch and Wikipedia (the latter only in cases where the information is not available in the previous sites) with their classification of a newspaper bias. We extract this information for newspapers from USA, Germany, Spain and Catalonia. With the list of newspapers, their URL,[4] and their stance, we use OSCAR, a multilingual corpus obtained by filtering the Common Crawl (Ortiz Suárez et al., 2019; Abadji et al., 2021), to retrieve the articles. Appendix A lists the sources used in this work: 47 USA newspapers with 742,691 articles, 12 German with 143,200, 38 Spanish with 301,825 and 19 Catalan with 70,496.
\
Topic Modelling. Not all articles have a bias, some topics are more prone than others. While the Sports section of a newspaper is usually less prone to reflect political biases, the opposite happens with the International section. We therefore use topics to select a subset of relevant training data for our binary classification. We do topic modelling on the articles extracted from OSCAR using Mallet (McCallum, 2002) which applies LDA with Gibbs sampling. We cluster the data in both 10 and 15 groups per language, roughly corresponding to the number of sections a newspaper has. The keywords extracted for each topic are listed in Appendix B. We choose articles that fall under the topics we label as International, Government, Law & Justice, Economy, Live Science/Ecology, and specific language-dependent topics such as Immigration and Violence for English, Nazism for German, and Social for Spanish. The selection is done after the inspection of the keywords. For the final dataset, we do the union of the selected articles clustered to 10 and 15 topics. The process filters out 49% of the Spanish articles, 39% of the German and 31% of the English ones.
\
Preprocessing and Cleaning. We discard articles with more than 2000 or less than 20 words before cleaning. Afterwards, we remove headers, footers and any boilerplate text detected. This text has the potential to mislead a neural classifier, as it might encourage the classifier to learn to distinguish among newspapers rather than focusing on their political stance. We select a newspaper per language and stance for testing and clean manually their articles. To create a balanced training corpus for each language, we randomly select a similar number of Left and Right-oriented articles from the remaining collection. This balanced dataset is divided into training and validation as shown in Table 1 (top rows).
\
ChatGPT/Bard Corpus. We create a multilingual dataset with 101 articles. For this, we define 101 subjects including housing prices, abortion, tobacco, Barak Obama, etc. and translate them manually into the 4 languages (see Appendix D). The subjects consider topics prone to have a political stance such as those related to feminism, capitalism, ecologism, technology, etc. We also include proper names of people in the 4 countries being considered, whose biography may differ depending on the political stance of the writer. These subjects are inserted into the template prompt (and its translations into German, Spanish and Catalan):[5] Write a newspaper article on [SUBJECT]en
\
\
We prompt ChatGPT (GPT-3.5-Turbo) five times using the same subjects across four time periods. We generate the dataset with ChatGPT versions of Feb 13 (v02), Mar 23 (v03), May 24 (v05) and Aug 3 (v08); we cover the 4 languages simultaneously only with the last two. ChatGPTv05 generates significantly longer texts than the other ones with an article-oriented structure with slots to be filled with the name of the author, date and/or city. Multilingual Bard was available later, and we prompt it twice during the same period as ChatGPTv8.[6] Table 1 shows the statistics for this corpus.
\
\
[4] This implies selecting all the articles that are under a domain name of a news outlet, whether they are news or not.
\
[5] More specific prompts did not lead to different styles for the first versions of ChatGPT, for the last one we added more information such as …without subheaders. to avoid excesive subsectioning and/or bullet points. Neither ChatGPT nor Bard did always follow properly the instruction. The dataset we provide includes the prompts we used.
\
[6] Prompted 14–21 August 2023 from Berlin for English and German and from Barcelona for Spanish and Catalan as, contrary to ChatGPT, the generation depends on the location.
Welcome to Billionaire Club Co LLC, your gateway to a brand-new social media experience! Sign up today and dive into over 10,000 fresh daily articles and videos curated just for your enjoyment. Enjoy the ad free experience, unlimited content interactions, and get that coveted blue check verification—all for just $1 a month!
Account Frozen
Your account is frozen. You can still view content but cannot interact with it.
Please go to your settings to update your account status.
Open Profile Settings