Implementing AI well means revisiting your enterprise architecture
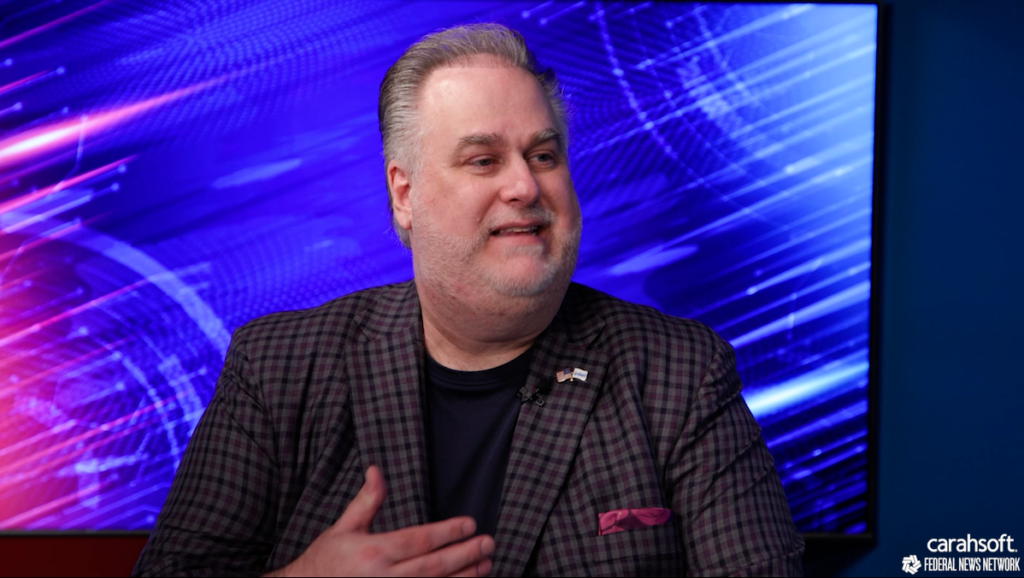
To many federal technology leaders, the rise of artificial intelligence, and now especially generative AI, will have a significant impact on their mission.
This means everything from automating routine tasks to helping mission area experts accelerate and improve their decision-making to advancing research and development more rapidly.
As with any technology, agencies need to continually consider the risks, challenges and security requirements of AI and the data it depends on.
Striking this right balance isn’t easy. Expert says it requires a systems-level approach that is both hardware and software centric and puts security, privacy and risk mitigation at the center of it all.
All of these facets of the AI journey have to lead to the responsible and safe use of AI.
Cameron Chehreh, the vice president and general manager for global public sector sales at Intel Corp., said for agencies to accelerate their safe and responsible use of AI, they have to focus on the age-old concept of enterprise architecture.
And before anyone sighs or tunes out, Chehreh said only by having a strong understanding of how your network, data and applications are set up can you answer the important questions to run AI tools:
Where is your data?
What data is in the cloud?
What data is still on-premise?
What’s the data that you want to use to feed or train models for AI?
Where do I need my data to best affect the user experience for AI?
“Enterprise architecture, whether in the civilian space or the defense architecture, that’s going to come into play again. We have to make sure we not only understand that to do what you talked about safety and security with AI, but also more importantly, where we’re going to stage the data assets and the algorithms to get us the best outcomes,” Chehreh said on the Innovation in Government show sponsored by Carahsoft. “It is fundamental to understand where do my systems reside to have the best experience for the end user because that’s really what it’s all about, and then being able to know where to stage those assets. If you remember, during the rise of the internet, we had things called content data distribution networks. Why did they come about? Well, they came about because the architecture and the data were so voluminous, we recognized at that point, to create a richer experience, at that time of data consumption, I had to pre-stage things in certain areas around the world. It’s the same thing here for agencies. They operate globally and nationally, so just having an architecture or a picture or a general understanding of where the data is going to sit, where the algorithm is going to sit and what sits between them because as we wander into the topic of security and safety, that becomes very important.”
The better the data, the better the AI
Agencies who can answer these and other questions will have an easier time creating knowledge or, as Chehreh referred to in Defense Department parlance, situational awareness.
He said while agencies have done a better job over the last decade identifying, protecting and taking advantage of their high-value data assets, many organizations still need to figure out how to use that data to train the AI models.
“More importantly, what are the lower quality data assets that they can start to really look at very differently? Do they actually need them on the network? Can they take them into a cold state, so that they can reduce the footprint of the attack surface?” Chehreh said. “The better quality input they have, the better quality output they are going to get, and AI is reminding us of that because it’s letting us almost look at ourselves in the mirror again, at this data and this information set, and begin to really formulate what is it we’re trying to train this new thing to go do for us in a very productive, high quality manner.”
Another challenge agencies have to begin get their arms around is a concept called sovereign AI.
Sovereign AI is the idea that organizations understand how the AI capabilities meet the policy and statutory requirements for countries, nations and governments.
Chehreh said while it’s still an emerging concept, it’s one agencies need to be aware of as they build out these tools.
“When we look at a global scale or if you’re in a cloud environment, data boundaries, data provenance, data security and data privacy all matter. So being able to build an AI solution in the public sector space becomes a little bit more challenging than, let’s say, if you’re in the commercial space because now what we’re talking about doing is we’re talking about cloud as an operating model versus a destination,” he said. “In that operating model, you now have to very clearly define the operational boundaries of what data is going to sit, what data it’s going to access and the access rights the AI will have to that data set on behalf of a sovereign entity. It becomes a little tricky.”
OT, IT convergence challenges
This is why the enterprise architecture discussion is, once again, at the heart of adopting new capabilities because agencies with that knowledge base can set boundaries and security requirements into their baseline infrastructure that will run the AI tools and capabilities.
“As you look at AI, it’s this interesting convergence between IT and operational technology. If I look at use cases, let’s say Postal Service, because they have sorting machines and sorting centers that have automation associated with them. If we augment them with AI, it can start preventing the machines from doing something unsafe,” he said. “As we talk about this convergence of the OT and IT, things like basic hygiene, encryption for data at rest and for data in motion, the use of good encryption keys are all part of this more dynamic versus static environment.”
Chehreh said as agencies continue their AI journeys, it’s clear they need to understand their data, their enterprise architecture and the rules that govern their entire network.
“It creates a blueprint for your success. It’s not about the plan for the [plan’s sake] in detail, but it’s creating that blueprint, knowing the journey you’re on, taking those first baby steps, and through those lessons learned that you’re finding, folding them back in rather quickly and be very agile about the process,” he said. “AI doesn’t have to be costly. Right now, there’s this association with AI and having to use accelerators and having to use other more advanced, very costly hardware, but what we’re finding in practicality is yes, for certain functions, when I have to train models and I need to do things fast, that absolutely requires exquisite hardware. Much too many people are surprised your existing infrastructure, whether it just be common CPU, has the ability now to be able to run a lot of the production capabilities that we’re talking about with AI so that you’re getting better scale.”
Listen to the full show:
<""""""""""""><><><><><><><><><><><><><><""><><><><><><><><><><><>
The post Implementing AI well means revisiting your enterprise architecture first appeared on Federal News Network.
Welcome to Billionaire Club Co LLC, your gateway to a brand-new social media experience! Sign up today and dive into over 10,000 fresh daily articles and videos curated just for your enjoyment. Enjoy the ad free experience, unlimited content interactions, and get that coveted blue check verification—all for just $1 a month!
Account Frozen
Your account is frozen. You can still view content but cannot interact with it.
Please go to your settings to update your account status.
Open Profile Settings